The European Medicines Agency's (EMA) provides an overview of its position on specific issues related to modelling and simulation in the form of questions and answers (Q&As).
Users should read the Q&As in conjunction with the relevant scientific guidelines.
The Committee for Medicinal Products for Human Use (CHMP) and the Paediatric Committee (PDCO) may seek the input of the Methodology Working Party (MWP) to address specific questions in relation to modelling and simulation.
The date after each question refers to when it was first published.
Paediatrics
The use of model informed drug development (MIDD) approaches in paediatric drug development is highly recommended due to the practical and ethical limitations in collecting experimental pharmacokinetic (PK)/ pharmacodynamic (PD)/clinical data in this group of patients and the potential of these approaches to quantify the effects of growth and maturation on Dose-ExposureResponse (DER) by leveraging data from literature and older patients.
The prediction/characterisation of PK and DER in children and neonates based on MIDD can serve as the basis for dose/regimen selection, clinical trial optimisation (e.g., using optimal sampling or simulation/estimation approaches), extrapolation [1,2], and posology claims in the summary of products characteristics (SmPC). MIDD approaches should adhere to the highest standards and regulatory guidance [3-7] especially when of high regulatory impact.
These approaches are heavily dependent on the disease area, availability of surrogate biomarkers and characteristics of clinical endpoints making any general recommendations very challenging.
The following Q&As will focus on PK prediction in children and neonates and its applications which is the area with the most MIDD approaches seen in paediatric investigation plans.
References
- E11(R1) Step 5 Clinical investigation of medicinal products in the paediatric population (europa.eu)
- Reflection paper on the use of extrapolation in the development of medicines for paediatrics - Final (europa.eu)
- Guideline on the reporting of physiologically based pharmacokinetic (PBPK) modelling and simulation- Final (europa.eu)
- Guideline on reporting the results of population pharmacokinetic analyses (europa.eu)
- ASME framework: Assessing Credibility of Computational Modeling through Verification and Validation: Application to Medical Devices, 2018.
- Skottheim Rusten I, Musuamba FT. Scientific and regulatory evaluation of empirical pharmacometric models: An application of the risk informed credibility assessment framework. CPT Pharmacometrics Syst Pharmacol. 2021 Nov;10(11):1281-1296. doi: 10.1002/psp4.12708. Epub 2021 Oct 8. PMID: 34514745; PMCID: PMC8592505.
- Musuamba FT, Skottheim Rusten I, Lesage R, Russo G, Bursi R, Emili L, Wangorsch G, Manolis E, Karlsson KE, Kulesza A, Courcelles E, Boissel JP, Rousseau CF, Voisin EM, Alessandrello R, Curado N, Dall'ara E, Rodriguez B, Pappalardo F, Geris L. Scientific and regulatory evaluation of mechanistic in silico drug and disease models in drug development: Building model credibility. CPT Pharmacometrics Syst Pharmacol. 2021 Aug;10(8):804-825. doi: 10.1002/psp4.12669. Epub 2021 Jul 13. PMID: 34102034; PMCID: PMC8376137.
The Q&A refers to cases where the aim of the pharmacokinetic (PK) studies and/or simulations will be to define dose regimens for paediatric patients targeting concentrations which have been shown to be effective and safe in adults. A prerequisite is that the therapeutic range, the relevant exposure metrics linked to efficacy and safety and the exposure response relationship are established in adults. Examples of relevant exposure metrics are area under the concentration-time curve, maximum concentration, or minimum concentration. The model that will serve as a basis for predictions needs to be adequate for the intended use.
Different presentations of the data should be available for regulatory decision making. In the following, some example figures are given to illustrate which aspects will be important for regulatory evaluation (e.g. in the context of a paediatric investigation plan, a scientific advice, or an application for marketing authorisation).
In general, the most relevant covariate to influence PK in paediatric patients is considered to be body weight accounting for size differences, in addition to age in the youngest paediatric patients to account for maturation of drug eliminating processes (see Question2). Therefore, the example plots are depicted with body weight and age as covariates but comparable figures will be needed if other covariates are considered necessary.
Relevant predefined exposure metrics should be presented graphically versus body weight and age on a continuous scale (example figure 1). In addition, if the drug is indicated to be used in the age range below one year, exposure versus body weight and age should be depicted in an additional separate figure focused on children 0 – 1 year of age.

If different doses are proposed for bands of weight or/and age, exposure ranges predicted for the proposed doses for the subsets of the paediatric population should be visualized as boxplots (example figure 2). The reference range in the adult population (median and outer percentiles of observed or simulated data) should be given additionally (example figure 2). The same statistics should be presented in numbers in tables.
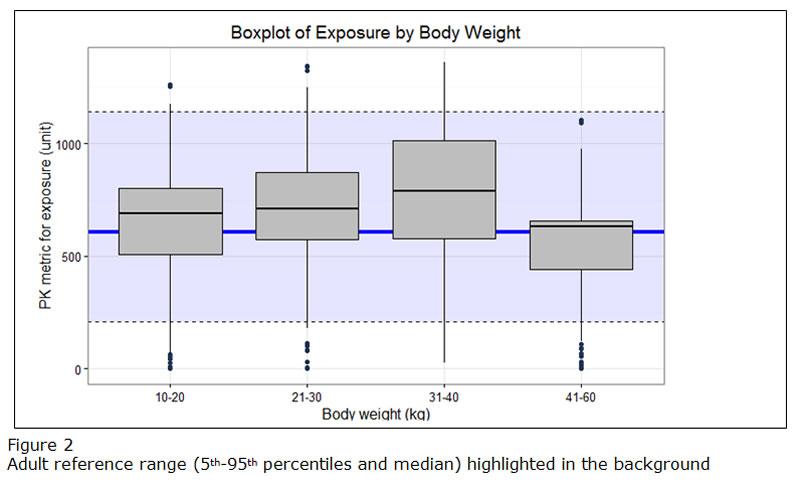
Overall, the recommended dosing regimen should follow as closely as possible the function describing the PK in paediatrics. To illustrate this, a figure comparing the proposed doses to the doses resulting from the underlying function (e.g. allometric scaling) should be provided (example figure 3). In this case, alternative 2 (the green line) is considered as the optimal dosing regimen.
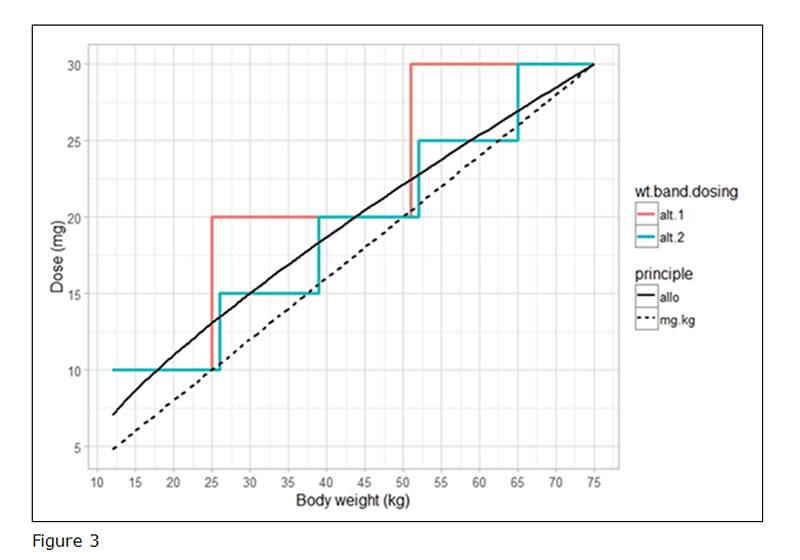
Reference
- Schoemaker, R. et al. Brivaracetam population pharmacokinetics in children with epilepsy aged 1 month to 16 years. Eur J Clin Pharmacol 2017; 73: 727–733.
It is important to include body weight relationships when describing pharmacokinetic (PK) in paediatric patients. The theoretical values of allometric exponents (0.75 for clearance and 1.0 for volume of distribution) are considered to have physiological basis [1], and often provide adequate explanation for body weight relationships in paediatric patients [2,3]. The exponents are often estimated and for different reasons these estimates may differ from the theoretical values. In neonates the maturation level of eliminating organs influences the estimates and in adults the body composition will affect them. It is often the case that the data generated in the context of a paediatric development program are too limited to conclude on whether the estimates of the allometric exponents are in fact different from the theoretical values. The selection of allometric scaling exponents should be based on the available information on the pharmacology of the drug and age of the target population, in conjunction with known properties of organ maturation [4]. In light of the theoretical framework, the MSWP considers the use of fixed exponents both scientifically justified and practical when developing population PK models in children however, regardless of whether estimated or fixed allometric exponents are used, the selected approach should be scientifically justified in the analysis plan. Further, it is not advised to use allometric exponents estimated using adult data for paediatric PK models, as adult exponents are likely to be affected by other factors than pure body size relations (such as obesity and model misspecifications).
As said, in lower ages there are also maturation functions that come into play, and for these paediatric patients (age justified by relevant organ ontogeny) it is important to include maturation function(s) to describe paediatric pharmacokinetics. Due to high correlation between body weight and age in paediatric patients, maturation functions and allometric exponents should not be estimated simultaneously [5]. To conclude, it is often a useful approach to used fixed allometric exponents when analysing paediatric data.
References
- Kleiber, M. Body size and metabolism. Hilgardia 1932; 6: 315–333.
- Mahmood I. Dosing in children: a critical review of the pharmacokinetic allometric scaling and modelling approaches in paediatric drug development and clinical settings. Clin Pharmacokinet 2014; 53: 327-46.
- Germovsek, E. et al. Scaling clearance in paediatric pharmacokinetics: All models are wrong, which are useful? B J Clin Pharmacology 2017; 83: 777-790.
- Holford N. et al. A pharmacokinetic standard for babies and adults. J Pharm Sci 2013; 102:2941-52.
- Bonate PL. The effect of collinearity on parameter estimates in nonlinear mixed effects models. Pharm Res 1999; 16(5): 709-17.
The inclusion of factors related to ontogeny or organ maturity are essential to ensure the accuracy of developed models. Where appropriate, factors associated to changes in growth (body weight and size, organ weight), organ function (gastrointestinal (GI) pH, GI volume, GI transit times, portal vein flow rates, cardiac output), tissue composition (plasma proteins, water and fat composition), clearance (renal [figure 1], biliary) or metabolic processes [Cytochrome P450s (CYPs) [figure 2], Uridine diphosphate-glucuronosyltransferase, (UGTs)]) should be accounted for in the model. The relevance of factors and therefore their inclusion in the model should be based on the pharmacology of the drug, the age and physiology of the target population and the dose and route of administration of the drug. The level of evidence required for a model (e.g. in vitro vs. in vivo CYP expression [1,2], comprehensive vs sparse pharmacokinetic (PK) data, etc) depends on whether it is used for descriptive purposes or for extrapolation. Nonetheless, models require the similarities and potential differences between source and target population to be described and assumptions in the model should be justified [3], and where appropriate, models should be qualified (i.e. for physiologically based pharmacokinetic (PBPK) models) if used to support labelling.

By its very nature, maturation is a time-dependent factor, and cannot be explained using allometric scaling. Significant changes in weight or by maturation either over the course of a study or the duration of treatment should be accounted for as appropriate. For example, changing body weight and maturation should be accounted for in a PK model where there is significant change during the treatment duration; this is especially true for premature neonates who undergo a catch up phase of growth, and as such, simply investigating baseline bodyweight in this sub-population may lead to erroneous conclusions. Maturation is generally described using a sigmoid Emax or Hill equation model, although linear or exponential models can be used [4]. Many PBPK modelling platforms include an ability to account for processes that are subject to ontogenic maturation. Where appropriate, maturation should be included in population PK modelling.
Care needs to be taken to bridge results from a specific (healthy) population to the target paediatric population, especially where disease progression would have an impact on maturation and ontogeny [1]. Where modelling is used to characterise dose-exposure-response relationships, developmental maturation and disease progression should also be taken into account e.g. neurological development for anti-epileptic drugs [5].
References
- Cole, S. et al. European regulatory perspective on pediatric physiologically based pharmacokinetic models. Int J Pharmacokinet 2017; 2(2): 113-124.
- Upreti, V. and Wahlstrom, J. Meta-analysis of hepatic cytochrome P450 ontogeny to underwrite the prediction of pediatric pharmacokinetics using physiologically based pharmacokinetic modelling. J Clin Pharmacology 2016 56(3): 266-283.
- European Medicines Agency. Draft reflection paper on the use of extrapolation in the development of medicines for paediatrics. 2017
- Holford, N. et al. A pharmacokinetic standard for babies and adults. J of Pharm Sci 2013; 102: 2941-2952.
- van Dijkman, S. et al. Pharmacotherapy in pediatric epilepsy: from trial and error to rational drug and dose selection – a long way to go. Expert Opinion on Drug Metabolism and Toxicology 2016; 12(10): 1143-1156.
- Lu, H. and Rosenbaum, S. J Pediatr Pharmacol Ther 2014 ; 19(4): 262–276.
Systems knowledge on PK in neonates should be considered when predicting PK. For example, developmental and environmental changes in the gastrointestinal tract (GIT) in newborns [1] are important for the oral absorption of drugs. Distribution of a drug can be affected by changes in body composition, such as changes in total body water and adipose tissue which is different in neonates compared to older children and adults. The ontogeny of liver enzymes and kidney should be considered when predicting PK in neonates. Plasma protein-binding and tissue-binding changes arising from changes in body composition with postnatal growth and development may also influence drug distribution. Maternal Ig transfer (placental and via milk) in neonates can also impact the pharmacokinetics of monoclonal antibodies.
No standard approach that fits all molecules and clinical scenarios can be recommended as the complexity in ADME is impacting the degree of uncertainty in model predictions down to the neonatal age. The PK of a drug for example renally excreted to a very high percentage, potentially without involvement of liver enzymes or transporters (e.g., monoclonal antibodies targeting an exogenous target [2]) and administered intravenously is predictable more reliably than the PK of a drug with more complex ADME and elimination pathways.
The views expressed below are based on previous regulatory experience with MIDD approaches for predicting neonatal PK, noting that especially for PK predictions in extremely low birth weight and preterm neonates, no experience is available in the regulatory system.
The two main MIDD approaches used for PK prediction in neonates are population PK modelling (popPK) and physiological based pharmacokinetic modelling (PBPK).
Population PK modelling (popPK)
The model is first developed/evaluated in adults followed by adaptations to describe PK in adolescents and paediatric patients and scaled to neonates using allometry with fixed theoretical exponents and published ontogeny functions. This approach is detailed in Q&As 2 & 3 above. In brief, published maturation functions for drugs with similar physicochemical/PK properties or maturation functions for the eliminating organs, are integrated in the population PK model established for older patients. For example, maturation of GFR, described by Rhodin et al. [3] has been implemented in population PK models for various compounds for describing the PK in (pre-) term neonates [4,5,6]. Recently a continuous model for renal function has been proposed [7]. These models require a thorough understanding/characterization of the compounds’ physicochemical characteristics and PK properties (enzyme/transporter affinity, main route of elimination, etc.) for selecting an appropriate (set of) maturation functions to integrate in the popPK model. No universal model or maturation function exist. The adequacy of the chosen model to predict the PK needs to be justified for each drug.
Physiological based pharmacokinetic modelling (PBPK)
A PBPK model is first developed and evaluated in adults, followed by adaptations to describe PK in adolescents and/or paediatric patients and scaled to neonates using mechanistic models accounting for growth and maturation in e.g., body composition, blood/lymph flows, organ function, enzyme/transporter activity.
The PBPK modelling approach provides a more mechanistic alternative to the population PK modelling approach outlined before. Its role in paediatric drug development programs has been reviewed by several authors [8,9,10]. The equations describing the physiological maturation functions appear platform-specific and/or specific to classes of drugs, and large-scale qualification is typically not available. Nevertheless, (platform-specific) meta-analyses are frequently published demonstrating the usefulness of the approach for predicting PK exposure in paediatric patients [11,12].
Limited information is available on the qualification of the different approaches for the prediction of PK exposure in the neonatal population. Publicly available, platform-independent data repositories, such as the “ontogeny knowledge base” advocated by the FDA [13] or data/model sharing initiatives such as the in silico clinical pharmacology backbone advocated by EMA [14], are critical to build confidence in the model predictions.
In both MIDD approaches sensitivity analyses and stochastic simulations should be conducted to explore the impact of the magnitude of the inter-individual variability and other model assumptions on the expected exposure distribution and central tendency across different sub-groups (e.g., stratified by age, body weight, concomitant medication, etc.). At present it is unclear whether the estimated magnitude of the inter-individual variability in adults/adolescents correlates with the inter-individual variability in neonates. Appropriate justification for the estimate of inter-individual variability as input for the simulations should be provided.
In the absence of qualified and platform-independent models for PK prediction in neonates, a pragmatic approach would be to conduct sensitivity analyses using different PBPK/popPK platforms, models, scaling, and maturation functions to account for model/parameter uncertainty when predicting PK in neonates.
Owing to the rapidly changing physiology in the neonatal population, a large uncertainty on the predictions is expected irrespective of the approach used. This is especially relevant in the context of narrow therapeutic index drugs and long-acting drugs. Therefore, well-designed PK studies remain necessary to characterise PK in neonates.
References
- Physiology of the Neonatal Gastrointestinal System, April Neal-Kluever, Jeffrey Fisher, Lawrence Grylack, Satoko Kakiuchi-Kiyota and Wendy Halpern Drug Metabolism and Disposition March 1, 2019, 47 (3) 296-313; DOI: https://doi.org/10.1124/dmd.118.084418
- Pharmacokinetics and clinical pharmacology of monoclonal antibodies in pediatric patients. ZH Temrikar, S Suryawanshi, B Meibohm. Pediatric Drugs 22 (2), 199-216
- Rhodin, Malin M., et al. "Human renal function maturation: a quantitative description using weight and postmenstrual age." Pediatric nephrology 24.1 (2009): 67-76.
- Wang J, Kumar SS, Sherwin CM, Ward R, Baer G, Burckart GJ, Wang Y, Yao LP. Renal Clearance in Newborns and Infants: Predictive Performance of Population-Based Modeling for Drug Development. Clin Pharmacol Ther. 2019 Jun;105(6):1462-1470. doi: 10.1002/cpt.1332. Epub 2019 Feb 10. PMID: 30565653; PMCID: PMC6513721.
- Johansson, Åsa M., et al. "A population pharmacokinetic/pharmacodynamic model of methotrexate and mucositis scores in osteosarcoma." Therapeutic drug monitoring 33.6 (2011): 711-718.
- Hennig, Stefanie, et al. "Population pharmacokinetics of tobramycin in patients with and without cystic fibrosis." Clinical pharmacokinetics 52.4 (2013): 289-301.
- O'Hanlon CJ, Holford N, Sumpter A, Al-Sallami HS. Consistent methods for fat-free mass, creatinine clearance, and glomerular filtration rate to describe renal function from neonates to adults. CPT Pharmacometrics Syst Pharmacol. 2023 Mar;12(3):401-412. doi: 10.1002/psp4.12924. Epub 2023 Feb 7. PMID: 36691877; PMCID: PMC10014044.
- Ince, Ibrahim, et al. "Predictive pediatric modeling and simulation using ontogeny information." The Journal of Clinical Pharmacology 59 (2019): S95-S103.
- Development and Application of a Physiologically-Based Pharmacokinetic Model to Predict the Pharmacokinetics of Therapeutic Proteins from Full-term Neonates to Adolescents. Pan X et al., AAPS J. 2020 May 24;22(4):76. doi: 10.1208/s12248-020-00460-1. PMID: 32449129.
- Bi, Youwei, et al. "Model?Informed Drug Development in Pediatric Dose Selection." The Journal of Clinical Pharmacology 61 (2021): S60-S69.
- Upreti, Vijay V., and Jan L. Wahlstrom. "Meta?analysis of hepatic cytochrome P450 ontogeny to underwrite the prediction of pediatric pharmacokinetics using physiologically based pharmacokinetic modeling." The Journal of Clinical Pharmacology 56.3 (2016): 266-283.
- Ince, Ibrahim, et al. "Predictive Performance of Physiology?Based Pharmacokinetic Dose Estimates for Pediatric Trials: Evaluation With 10 Bayer Small?Molecule Compounds in Children." The Journal of Clinical Pharmacology 61 (2021): S70-S82.
- Burckart GJ, Seo S, Pawlyk AC, et al. Scientific and Regulatory Considerations for an Ontogeny Knowledge Base for Pediatric Clinical Pharmacology. Clin Pharmacol Ther. 2020;107(4):707-709. doi:10.1002/cpt.1763
- Manolis, E., Musuamba, F.T. and Karlsson, K.E. (2020), Regulatory Considerations for Building an In Silico Clinical Pharmacology Backbone by 2030. Clin. Pharmacol. Ther., 107: 746-748. https://doi.org/10.1002/cpt.1772
The goals of PK studies should be to investigate PK and safety in neonates. For PK a realistic objective would be to exclude clinically relevant violations of assumptions underlying the MIDD approach and clinically relevant over or under prediction of PK in neonates. If relevant and feasible, exposure response analyses (informed by adult & literature data) should justify the appropriate PK metrics and associated target precision.
To achieve these goals, PK studies should be adequately designed with optimised sample sizes and sampling schedules. Implementation of adaptations such as interim analyses for PK evaluation or therapeutic drug monitoring should be considered. MIDD should be used to justify critical design features (number of samples, number of patients, interim analyses etc.) and to inform on the efficiency/feasibility of the proposed trial design and expected parameter precision prior to patient inclusion. Deviations from the MIDD approach should be duly justified.
Partial replacement of clinical PK data is a common scenario where PK in neonates is characterized by sparse PK sampling and popPK or/and PBPK models.
Due to the high degree of uncertainty in PK prediction/characterisation in neonates, a full PK waiver is not recommended from a methodological point of view. Nevertheless, the clinical reality such as trial feasibility, safety and unmet medical need is considered when deciding on the PK study requirements.
Established systems knowledge (e.g., as in the case of renal function maturation for predominately renally excreted drugs) could be considered as an additional argument in favour of a full replacement of a PK study in neonates considering also clinical factors as discussed above. So far, only one example could be found where full replacement was deemed justified (dose selection of gadolinium-based contrast agents in infants and neonates [1]).
In case of full replacement of PK studies (i.e., high regulatory impact), the MIDD approaches should adhere to the highest standards and will be subject to high degree of regulatory scrutiny.
References
- US Food and Drug Administration (FDA). FDA clinical pharmacology review of Multihance. Published 2017
Dose selection in neonates should be based on the established or assumed DER in this group. In this context MIDD approaches are highly recommended [1,2].
For de novo characterization of DER, and subject to clinical judgement, dose ranging studies in neonates would be needed. However, in some situations and depending on the extrapolation concept, the DER characterized in adults or older children can be extrapolated to neonates. MIDD approaches can support this extrapolation by accounting for the organ maturation effects on DER.
Assuming a similar ER relationship as in older children and adults, dose selection could be based on exposure-matching. Adequate characterisation of the PK in neonates is key in this regard (see Q&A5 and Q&A6). For presentation of results/simulations to aid dose selection in neonates, please refer to Q&A1.
When pharmacodynamics is expected to be altered in neonates, the selection of dose in neonates should be based on PK/PD simulations. Uncertainties will be even higher with PK/PD predictions than those associated with PK prediction, which should be factored in when designing a clinical study in neonates (e.g., need for interim analysis, dose ranging).
PopPK(/PD) and PBPK(/PD) models as discussed in Q4 (with the addition of PD component, if needed) are acceptable methods in this setting. Other mechanistic models such as Quantitative Systems Pharmacology approaches could be also considered [3] but, to date, there is limited experience of such models in the European regulatory network.
References
- The European Medicines Agency Experience With Pediatric Dose Selection, Efthymios Manolis, Flora T.Musuamba, and Kristin E. Karlsson. The Journal of Clinical Pharmacology 2021, 61(S1) S22–S27
- General Clinical Pharmacology Considerations for Neonatal Studies for Drugs and Biological Products Guidance for Industry. FDA
- Azer K, Barrett JS. Quantitative system pharmacology as a legitimate approach to examine extrapolation strategies used to support pediatric drug development. CPT Pharmacometrics Syst Pharmacol. 2022 Jul;11(7):797-804. doi: 10.1002/psp4.12801. Epub 2022 May 24. PMID: 35411657; PMCID: PMC9286717.
Model-based approaches for approval of alternative dosing regimens and routes of administration of (anti PD-1 and PD-L1) monoclonal antibodies
Recently, various posology changes for monoclonal antibodies such as change from body weight-based to flat dosing, change in dosing interval or in route of administration (and related formulation) relying on Model Informed Drug Development (MIDD) approaches have been issued as type II variations or line extensions. Moreover, there have been repeated requests directed to the scientific advice working party regarding the acceptability of model-based approaches for approval of new routes of administration (and related formulations) and posology of (approved) monoclonal antibodies. In particular, there have been several requests relating to anti-PD-1/PD-L1 monoclonal antibodies (mAbs). The purpose of this Q&A is to provide clarity on the acceptability of such approaches. The focus is on anti-PD-1/PD-L1 mAbs but the general principle applies to other mAbs as well.
The questions-and answers below address situations when modelling and simulations of pharmacokinetics (PK) and dose-exposure-response (D-E-R) relationships for efficacy and safety with or without additional clinical studies, can be acceptable to support such changes.
Model-based approaches are acceptable as the main source of evidence to inform approval of alternative posology or route of administration for mAbs, including PD1/PDL-1 inhibitors. For this purpose, modelling and simulation analysis should be adequately implemented [1] to characterize the PK metric(s) (minimum concentration (Cmin), area under the concentration-time curve within a dosing interval at steady state (AUCtau,ss), area under the concentration-time curve after first cycle or maximum concentration (Cmax))) of interest as well as their link with efficacy and safety. The data collection should be optimized to ensure that the data used to develop and validate the models are of acceptable quality and informative to address the question of interest. Methods used for simulations should also be based on the highest standards. Graphical (e.g. comparison of boxplots) and numerical analyses (e.g. non-inferiority testing, comparison of proportions of simulated patients above and below critical concentration values related to efficacy or safety, etc.) should be used to support the approval of the new route of administration or posology.
Relevant data/information should be used for D-E-R analysis in order to adequately characterize the link between the dosing and clinical outcomes given the complex relationships between PK and pharmacodynamics (PD). Relevant data for PK e.g. free and total drug concentrations in plasma (measured using appropriate bioanalytical techniques) and their immunogenic potential should be considered. As regards pharmacodynamic and clinical response, different markers and outcomes can be informative for establishing exposure-response relationships. Pharmacodynamic markers include receptor expression, receptor occupancy (e.g. measured on immune cells in blood and/or in tissue), effector to target cell ratio, presence of soluble target engagement, the formation of a trimolecular complex between the drug, T-cell and tumour cell as well as biomarkers (e.g. dynamic measurement of multivariable ‘signatures’). Informative clinical activity outcomes include objective response rate (ORR) or tumour burden dynamics measurements, and/or time-dependent efficacy measures, such as progression free survival (PFS)/overall survival (OS) and safety outcomes such as early and delayed immune-related adverse events. In addition to landmark analyses, dynamic changes over time for both exposure and response measurements can be considered for establishment of reliable exposure-response relationships.
It should be noted that dose ranging studies, including more than one dose regimen, are often needed to establish a robust exposure-response relationship for anti-PD-1/PD-L1 antibodies in cancer indications (in particular because of the confounding effects of patient disease/health status on the mAb PK).
It is important that the modelling approaches used for D-E-R analysis are suitable to the available data/knowledge and relevant to the question to be addressed. Particular attention should be given to factors that may contribute to variability in the results, such as the type of in vitro assays and experimental settings used to measure the pharmacodynamic biomarkers, and to the link between pharmacodynamic biomarkers and relevant clinical efficacy endpoints. Any kind of bracketing or worst-case scenario approach should be informed by appropriately conducted D-E-R analysis.
Specific topics that need to be considered for new routes of administration (and related pharmaceutical formulations) of approved anti-PD-1/PD-L1 mAbs include differences in PK profiles, immunogenicity effects and other safety issues related to each formulation.
As a general principle, worsening of efficacy and safety should be excluded with the new routes of administration (and related formulations). This is to ensure that there is no change in the benefit/risk profile of the concerned mAb. If D-E-R is well established for the concerned mAb, the ranges of values of clinically relevant PK and/or pharmacodynamic metrics leading to the efficacy and safety levels for which positive benefit/risk has been established should be known. Therefore, if the PK metrics with the new routes of administration (and related pharmaceutical formulations) are maintained within these ranges, they can be considered similar to the corresponding metrics with the approved route of administration (and related pharmaceutical formulations). Model-based approaches can be used to characterize D-E-R and to demonstrate the similarity between the PK- or pharmacodynamic metrics.
For example, a subcutaneous (SC) formulation can be proposed as a follow-up to an approved intravenous (IV) formulation of an anti-PD-1/PD-L1 mAb within the same indication. In this case, the relevant PK metrics (such as Cmin, AUCt,ss, AUC after the first cycle or Cmax) should prove similar to the exposure reached with the approved IV formulation.
The relevance of the PK parameter(s) and metric(s) for the efficacy and safety and the acceptance criteria for PK comparability should be adequately justified based on exposure-response considerations. Therefore, PK similarity should be demonstrated for central tendency (e.g. median, geometric mean, etc.) and dispersion parameters (range, prediction intervals, etc.), respectively.
Success criteria should be predefined in the protocol/analysis plan.
Of note, if a new route of administration (and related formulation) is proposed, additional clinical studies should be performed in line with the recommendations in the relevant EU guidelines [2]
The PK exposure (i.e. relevant PK metrics such as Cmin, AUC or Cmax) will need to prove similar to the exposure reached with the dosing regimen for which positive benefit/risk has been established. The relevance of the PK parameter/metric and the acceptance criteria for PK comparability (as described in the answer to Q2) for the efficacy and safety should be adequately justified based on established exposure-response relationship. Because of the time-varying clearance in response to treatment, pharmacokinetic data of the first/earlier dose(s) should also be considered. The PK similarity or less variability for the alternative dosing regimen should be demonstrated for central tendency (e.g. median, geometric mean, etc.) and dispersion parameters (range, prediction intervals, etc.), respectively.
Depending on the type of change in dosing regimen, different aspects need to be considered:
- Case 1: Change from body weight-based dosing to a flat dose:
In this case it is expected that there will be a shift in the subpopulation (body weight range) that will have an extremely high/low exposure. It is important to provide exposure comparisons across the full body weight range for the population to ensure that the expected exposure range with the flat dose is acceptable for all weight groups.
- Case 2: Change in dosing interval:
When changing the dosing interval, e.g. from every other-week to every four-week dosing, the PK profile of the drug will change. Exposure-response considerations should be the basis for decision-making regarding the clinical relevance of the difference in the shape of PK profile for efficacy and safety. Longitudinal PK/PD (target engagement, tumor size, other biomarkers) modelling could provide supporting information that the changes in PK profile are not associated with changes in the benefit/risk profile of the alternative dosing regimen.
The extrapolation refers to establishing the PK or pharmacodynamic similarity (as defined in the answer to Q2). This will cover alternative routes of administration (e.g. SC vs. IV) or dosing regimens (flat vs bodyweight-based dosing, change in dosing interval (e.g. weekly vs monthly)) in one indication and considering them comparable across indications.
Adequate characterization of the PK, PD and exposure-response relationship of the mAb as well as their determinants (covariates) in all the indications of interest is a prerequisite for the acceptability of extrapolation of posology, or alternative routes of administration across approved indications. Distribution of clinically relevant covariates in the model-building dataset and adequate covariate modelling across indications are considered of particular interest.
Differences in PK, PD and/or exposure-response that could preclude extrapolation of posology changes and alternative routes of administration across indications should be investigated. For example, the relevance of clinical endpoints (PFS/OS) may not be similar across indications/histologies. Moreover, because of the disease effects on PK, pharmacokinetic metrics might also vary across indications/histologies e.g. (neo)adjuvant and advanced cancer treatments.
Three different case scenarios are to be distinguished in this regard:
- Case 1: The PK exposures reached with the approved therapeutic doses/regimens in the concerned indication are known to be in the flat part of the exposure-response curve:
In this case, for efficacy, posology changes across indications based on PK comparability should be supported by simulations that should demonstrate non-inferiority of the relevant PK parameter/metric (Cmin, AUC) when compared to the corresponding metric with the approved dose regimen.
Non-inferiority margins and relevance of the chosen PK parameter/metric should be justified by exposure-response considerations and specified in the protocol/analysis plan.
If exposure-response is characterized for efficacy, but not for safety, a description of the safety profile of the mAb in the approved posology in the concerned indication should be included and justification should be provided to support a comparable or favourable safety profile with the alternative posology in the concerned indication.
- Case 2: The PK exposures reached with the approved therapeutic doses/regimens in the concerned indication are known to be in the ascending part of the exposure-response curve
In this case, posology changes across indications based on PK comparability should be supported by simulations, that should support PK similarity of the relevant PK parameter/metrics (Cmin, AUC) with the approved dosing regimen(s). The PK comparability for the alternative dosing protocol should be demonstrated for central tendency (e.g., median, geometric mean, etc.). In addition, comparability should be demonstrated for dispersion parameters (range, prediction intervals, etc.).
The relevance of the PK parameter/metric for the efficacy and safety should be adequately justified based on exposure-response considerations. Acceptance criteria for PK comparability should be specified in the protocol/analysis plan.
Longitudinal PK/pharmacodynamic (target engagement, tumor size other biomarkers) modelling could be useful in providing supporting information that the changes in PK profile are not associated with changes in the benefit-risk profile of the mAb in the concerned indication.
If exposure-response is characterized for efficacy, but not for safety, a description of the safety profile of the mAb in the approved posology in the concerned indication should be included and justification should be provided to support a comparable or favourable safety profile with the alternative posology in the concerned indication.
- Case 3: The exposure-response is not characterised in the concerned indication
Posology changes cannot be extrapolated across indications without understanding of the exposure response relationship.
Non-inferiority to the approved dose regimen should be demonstrated for drug activity such as ORR as primary endpoint, with similarity/non-inferiority of relevant PK parameters as secondary endpoints. Acceptance criteria for PK similarity/non-inferiority should be specified in the study protocol.
If exposure-response is not characterized for safety, a description of the safety profile of the approved posology in the concerned indication should be provided to support a comparable or favourable safety profile of the mAb in the alternative posology in the concerned indication.
Retrospective analysis of previously generated data is acceptable. The evidence required depends on the exposure range covered with alternative dosing regimens or routes of administration, the robustness of understanding of the PK and exposure-response of the mAbs and the quality of the modelling and simulation exercise.
It is essential that the data (e.g., data/models) used for (population PK or exposure-response) modelling and simulation are of acceptable quality and are informative for the corresponding analysis.
Success criteria should be specified in the protocol/analysis plan.